March 24, 2022
Danfoss drives are in all industrial processes. With drive-internal sensors they can detect important characteristics of customer processes. AC drive as a sensor offers accurate insights into customer applications and processes. This allows to develop digital services to optimize the processes. What is important to notice is that the scope is so much wider than just the drive – it is the entire customer application. Effective condition monitoring and early fault detection and diagnosis is critical for maintaining reliable operation, avoiding unpredicted breakdowns, reducing operating costs and improving productivity.
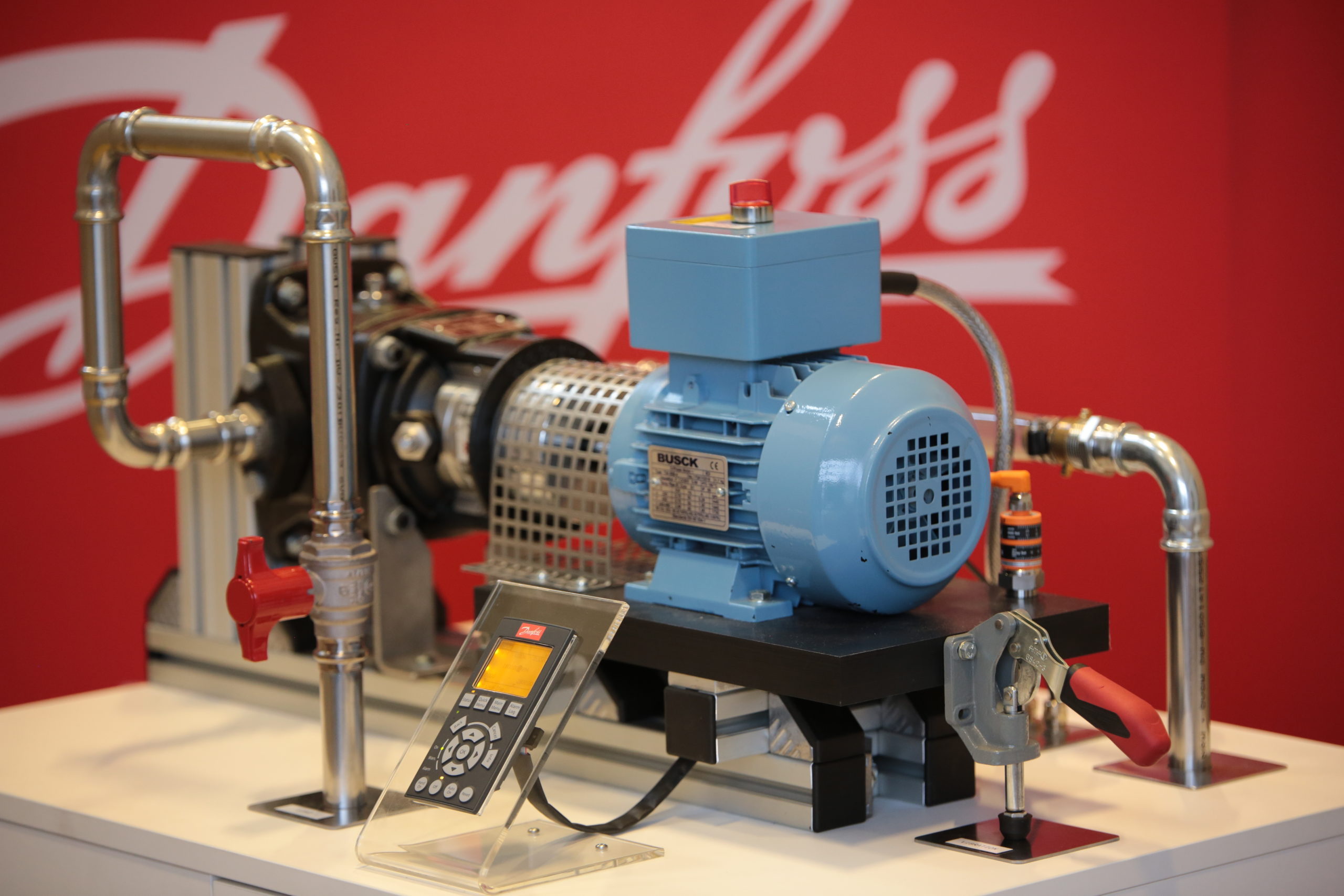
AC drive as a sensor offers accurate insights into customer applications and processes. Image: Danfoss
Many of the anomalies seen in industrial machines and processes are application specific. However, issues related to supply grid, AC drive and the motor being controlled are rather generic. With motor common issues relate to leak currents, wiring and bearings. With Tampere University, Danfoss has been looking at more efficient ways of detecting bearing faults. Bearing faults have the highest frequency of occurrence and are one of the most challenging to detect and diagnose.
Several different techniques have been applied to detect bearing faults. Recently data-driven deep learning models developed by the machine learning researchers have been proposed as solution. In earlier experiments these achieve very high classification accuracy. Unfortunately, their application carries significant cost. Complicated learning phase requires large, labeled data sets. Deep models have high computational complexity and cannot be applied in real time. The aim was at early fault diagnosis with scarce data and minimized neural network complexity to support real-time fault classification.
Based on this study we recommend using one-dimensional self-organized operation neural network with the generative neuron model for bearing fault severity classification, see Fig. 1. To keep the results comparable with earlier efforts we used commonly available data set, Fig. 2. The comparison shows that this technique is especially good in differentiating medium and severe faults. Computational complexity is low enough for real-time application. With the GPU implementation used in this experiment the total classification time was 9 µsec, see Fig. 3. The results have been published in IEEE Access and Mechanical Systems and Signal Processing [1]. The next step is to test this with the data collected from Danfoss drives.
The InDEx program is digitizing industry
DIMECC’s InDEx (Industrial Data Excellence) program focuses on digitizing Finnish industry and building a data community and a common data platform. Konecranes, Danfoss, Elekmerk, Fastems, HT Laser, PrimaPower, Raute, SSAB and TietoEVRY participate in the program. Academic partners are Aalto University, Tampere University, University of Turku, University of Helsinki, University of Jyväskylä and VTT. The InDEx program is co-financed by Business Finland and the participating companies. It is an Finnish Advanced Manufacturing Network (FAMN) development project.
[1] Turker Ince, Junaid Malik, Ozer Can Devecioglu, Serkan Kiranyaz, Onur Avci, Levent Eren, Moncef Gabbouj, “Early Bearing Fault Diagnosis of Rotating Machinery by 1D Self-Organized Operational Neural Networks,” IEEE Access, 2021. https://ieeexplore.ieee.org/abstract/document/9558835